AIOps Cloud Monitoring: Enhancing IT Operations with Intelligent Automation
Cloud monitoring has evolved rapidly in recent years, with artificial intelligence becoming a crucial component. AIOps, or Artificial Intelligence for IT Operations, represents the next frontier in managing complex cloud infrastructures.
AIOps platforms leverage machine learning and big data analytics to automate and enhance IT operations, providing real-time insights and predictive capabilities. These advanced systems can detect anomalies, identify root causes, and even implement corrective actions without human intervention.
The integration of AIOps with cloud monitoring is transforming how organizations approach digital transformation. By combining traditional monitoring techniques with AI-driven analysis, businesses can achieve unprecedented levels of efficiency and reliability in their IT operations. This proactive approach enables companies to anticipate and prevent issues before they impact users or customers.
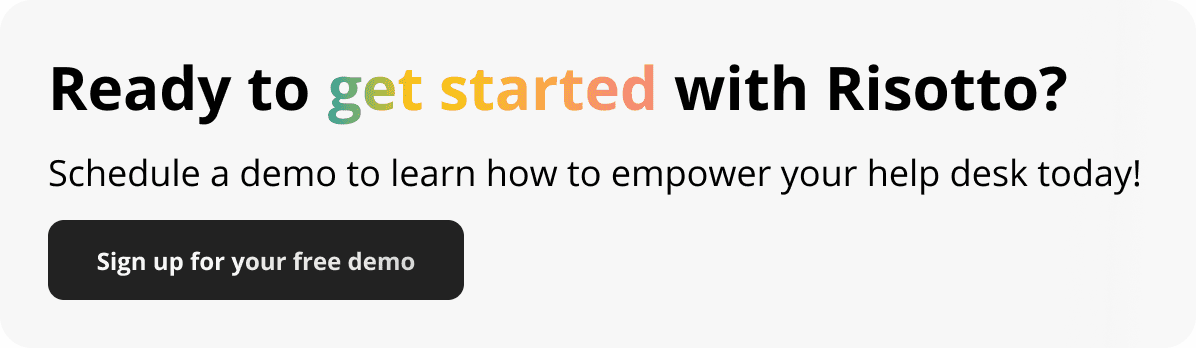
Understanding AIOPs and Cloud Monitoring
AIOps and cloud monitoring represent significant advancements in IT operations. These technologies leverage artificial intelligence and machine learning to enhance infrastructure management and service delivery in cloud environments.
Evolution of IT Operations
Traditional IT operations relied heavily on manual processes and reactive troubleshooting. As infrastructure grew more complex, this approach became unsustainable. Cloud migration introduced new challenges, requiring faster response times and greater scalability.
The rise of big data and machine learning paved the way for more sophisticated monitoring tools. These innovations led to the development of AIOps, which combines AI and IT operations to automate and streamline processes.
AIOps platforms analyze vast amounts of data from various sources, enabling proactive issue detection and resolution. This shift has transformed IT operations from a reactive to a predictive model.
Defining AIOps
AIOps stands for Artificial Intelligence for IT Operations. It integrates machine learning and big data analytics to automate IT operational processes. AIOps platforms collect and analyze data from multiple sources across an organization's IT infrastructure.
These systems use advanced algorithms to identify patterns, anomalies, and potential issues. By doing so, they can predict and prevent problems before they impact business operations.
Key components of AIOps include:
Real-time data ingestion and processing
Pattern recognition and anomaly detection
Automated incident management
Predictive analytics
Benefits of AIOps in Cloud Environments
AIOps offers numerous advantages for organizations managing cloud infrastructure. It enhances operational efficiency by automating routine tasks and reducing manual interventions.
Improved incident response is a significant benefit. AIOps systems can detect and diagnose issues faster than human operators, minimizing downtime and service disruptions.
Predictive analytics enable proactive maintenance, helping teams address potential problems before they escalate. This capability is particularly valuable in complex cloud environments where issues can quickly cascade.
AIOps also supports better decision-making by providing data-driven insights. IT teams can optimize resource allocation, improve capacity planning, and enhance overall system performance.
Cost reduction is another key advantage. By automating processes and improving efficiency, AIOps can significantly lower operational expenses associated with cloud management.
Key Components of AIOPs Platforms
AIOps platforms integrate several critical elements to enable effective cloud monitoring and management. These components work together to provide comprehensive insights and automated responses.
Data Management and Processing
AIOps platforms collect and process vast amounts of data from various sources. This includes metrics, logs, and traces from infrastructure, applications, and network devices. Advanced data ingestion techniques ensure real-time data collection and storage.
Data normalization and enrichment are crucial steps in the processing pipeline. These techniques help standardize data formats and add context to raw information. Machine learning algorithms then analyze this processed data to identify patterns and anomalies.
Scalable data storage solutions, such as distributed databases or data lakes, form the backbone of AIOps platforms. These systems can handle petabytes of data while maintaining quick access times for analysis.
Real-Time Analytics
AIOps platforms leverage advanced analytics to provide instant insights into system performance. Machine learning models continuously analyze incoming data streams to detect anomalies and predict potential issues.
Correlation engines identify relationships between different data points, helping to pinpoint root causes of problems. This enables faster troubleshooting and reduces mean time to resolution (MTTR).
Visualization tools present complex data in easily digestible formats. Interactive dashboards and customizable alerts help IT teams quickly grasp system status and respond to emerging issues.
Automation and Remediation
Automation is a key feature of AIOps platforms, enabling rapid response to detected issues. Predefined playbooks trigger automated actions based on specific conditions or thresholds.
These platforms can automatically scale resources, restart services, or apply patches without human intervention. This reduces manual workload and minimizes downtime.
AI-driven decision support systems suggest remediation steps to IT teams for complex issues. These recommendations are based on historical data and best practices, improving overall incident response efficiency.
Continuous learning algorithms refine automation rules over time, adapting to changing environments and improving accuracy in issue detection and resolution.
Challenges in Cloud Monitoring
Cloud monitoring faces several hurdles in today's complex IT environments. Organizations must navigate multicloud and hybrid infrastructures while maintaining visibility and integrating diverse data sources.
Complexities of Multicloud and Hybrid Cloud Systems
Multicloud and hybrid cloud architectures present unique monitoring challenges. Different cloud providers use distinct APIs, metrics, and management tools. This diversity complicates data collection and analysis across platforms.
Monitoring teams must adapt to varying service models and deployment options. They need to track resources spread across on-premises data centers and multiple cloud environments. This distributed nature makes it difficult to maintain consistent visibility and performance standards.
Security and compliance requirements add another layer of complexity. Each cloud provider has its own security controls and compliance certifications. Ensuring uniform security monitoring across these diverse systems requires specialized tools and expertise.
Ensuring Visibility across Disparate Systems
Achieving end-to-end visibility in cloud environments is challenging. Applications often span multiple services and infrastructure components. Tracing requests and transactions across these boundaries can be complex.
Cloud services may operate in isolated networks or behind firewalls. This isolation can limit access to performance data and logs. Monitoring teams must find ways to collect telemetry from these restricted environments without compromising security.
Dynamic scaling and ephemeral resources further complicate visibility efforts. Containers and serverless functions may exist for only short periods. Capturing meaningful data from these transient resources requires specialized monitoring approaches.
Integration of Different Data Sources
Cloud environments generate vast amounts of data from various sources. Logs, metrics, traces, and events all provide valuable insights. Integrating these disparate data types into a coherent monitoring solution is challenging.
Data formats and schemas often vary between cloud providers and services. Normalizing this data for consistent analysis requires significant effort. Monitoring tools must be flexible enough to handle diverse data structures and semantics.
Real-time data processing adds another layer of complexity. Cloud applications generate high volumes of telemetry data at rapid rates. Monitoring systems must efficiently ingest, process, and analyze this data to provide timely insights.
Correlating data across different sources is crucial for effective troubleshooting. Connecting application logs with infrastructure metrics and user experience data helps identify root causes. Building these correlations across diverse data sources remains a significant challenge in cloud monitoring.
Leveraging AI for Enhanced IT Operations
Artificial intelligence is transforming IT operations through advanced analytics, automated incident response, and intelligent troubleshooting. These AI-powered capabilities enable organizations to optimize their IT infrastructure and resolve issues more efficiently.
Predictive Analytics and Machine Learning Models
AI-driven predictive analytics uses historical data to forecast potential IT issues before they occur. Machine learning models analyze patterns in system logs, performance metrics, and other data sources to identify anomalies and predict future problems.
These models can detect early warning signs of impending failures or capacity constraints. This allows IT teams to take proactive measures, such as scaling resources or performing preventive maintenance.
Predictive analytics also enables more accurate capacity planning and resource allocation. By forecasting future demand, organizations can optimize their infrastructure and avoid over-provisioning or under-provisioning of resources.
AIOps for Proactive Incident Management
AIOps platforms leverage AI to streamline and automate incident management processes. These tools use machine learning algorithms to correlate alerts, identify the root cause of issues, and recommend remediation actions.
AI-powered incident management systems can automatically categorize and prioritize incidents based on their potential impact. This ensures that critical issues receive immediate attention from the appropriate teams.
AIOps tools also facilitate faster incident resolution through automated diagnostics and guided troubleshooting. By analyzing historical incident data, these systems can suggest effective solutions based on past resolutions.
Root Cause Analysis and Service Management
AI enhances root cause analysis by quickly sifting through vast amounts of data to identify the underlying causes of IT issues. Machine learning algorithms can detect complex relationships and dependencies that may not be apparent to human analysts.
AI-driven root cause analysis tools provide visual representations of problem areas and affected components. This helps IT teams understand the full scope of an issue and its impact on services.
In service management, AI improves ticket routing and classification. Natural language processing enables automated analysis of service requests, ensuring they are directed to the right support teams. AI can also suggest knowledge base articles and solutions based on ticket content.
Best Practices for Implementing AIOPs in Cloud Monitoring
Successful AIOps implementation in cloud monitoring requires careful planning and execution. Key factors include choosing appropriate tools, fostering team collaboration, and continuously improving AI models.
Selecting the Right AIOPs Tools and Platforms
When selecting AIOps tools and platforms, prioritize solutions that integrate seamlessly with existing cloud infrastructure. Look for platforms offering comprehensive monitoring capabilities, including real-time data analysis and predictive analytics.
Consider scalability to accommodate growing data volumes and evolving cloud environments. Evaluate the platform's ability to handle multi-cloud and hybrid setups.
Assess the tool's machine learning capabilities. Ensure it can effectively process and analyze diverse data types, including logs, metrics, and traces.
Choose platforms with robust automation features for incident response and remediation. This helps reduce manual workload and accelerates problem resolution.
Fostering Collaboration between IT Teams and AIOPs
Successful AIOps implementation requires close collaboration between IT teams and AIOps specialists. Establish clear communication channels and workflows to facilitate information sharing.
Create cross-functional teams that combine domain expertise with AI knowledge. This approach ensures AIOps solutions address specific operational needs.
Implement regular training sessions to familiarize IT staff with AIOps concepts and tools. Encourage hands-on experience to build confidence in using the new technologies.
Develop a feedback loop where IT teams provide insights to refine AIOps models and improve accuracy. This collaborative approach enhances the overall effectiveness of cloud monitoring.
Continuous Training and Fine-Tuning of AI Models
AI models in AIOps require ongoing training and fine-tuning to maintain accuracy and relevance. Establish a process for regularly updating models with new data to improve their predictive capabilities.
Implement a system for validating model outputs and performance. Use metrics such as false positive rates and prediction accuracy to assess effectiveness.
Adapt models to account for changes in cloud infrastructure or application behavior. This ensures AIOps remains effective as the environment evolves.
Leverage transfer learning techniques to apply knowledge from one domain to another, reducing training time for new use cases.
Regularly review and adjust model parameters based on real-world performance. This iterative approach helps optimize AIOps effectiveness in cloud monitoring over time.
Frequently Asked Questions
AIOps revolutionizes cloud monitoring by enhancing capabilities, streamlining processes, and leveraging machine learning. It offers improved incident response and adaptability across diverse cloud infrastructures.
How does AIOps enhance cloud monitoring capabilities?
AIOps enhances cloud monitoring by automating data collection and analysis. It provides real-time insights into system performance and potential issues.
AIOps tools can detect patterns and anomalies that human operators might miss. This leads to faster problem identification and resolution in cloud environments.
What are the primary functions of AIOps in cloud environments?
AIOps in cloud environments focuses on performance monitoring and optimization. It continuously analyzes metrics and logs to identify trends and potential bottlenecks.
Another key function is automated incident management. AIOps systems can detect, classify, and often resolve issues without human intervention.
Capacity planning is also improved through AI-driven predictive analytics. This helps organizations allocate resources more efficiently.
Which AIOps tools are best suited for cloud monitoring?
Prominent AIOps tools for cloud monitoring include Dynatrace, Splunk, and Datadog. These platforms offer comprehensive monitoring and analytics capabilities.
IBM Watson AIOps and Moogsoft are also popular choices. They excel in anomaly detection and automated incident response.
For open-source options, Prometheus paired with Grafana provides powerful monitoring and visualization features.
How does AIOps integration improve incident response in cloud computing?
AIOps integration significantly reduces mean time to resolution (MTTR) for cloud incidents. It achieves this by automating the incident detection and triage process.
AI-powered systems can correlate events across multiple data sources. This helps pinpoint root causes more quickly than manual analysis.
AIOps tools often include automated remediation features. These can implement fixes for common issues without human intervention.
What role does machine learning play in AIOps for cloud monitoring?
Machine learning is central to AIOps' predictive capabilities in cloud monitoring. It analyzes historical data to forecast future performance and potential issues.
ML algorithms can identify complex patterns in system behavior. This enables more accurate anomaly detection and proactive problem-solving.
Machine learning also drives continuous improvement in AIOps systems. They learn from each incident to refine their detection and response capabilities.
How do AIOps platforms differ in managing hybrid and multi-cloud infrastructures?
AIOps platforms for hybrid and multi-cloud environments offer unified monitoring across diverse infrastructures. They provide a single pane of glass for managing resources in different cloud platforms.
These tools typically include robust data integration capabilities. This allows them to collect and analyze metrics from various cloud providers and on-premises systems.
Advanced AIOps platforms can optimize workload placement across hybrid environments. They consider factors like cost, performance, and compliance requirements.
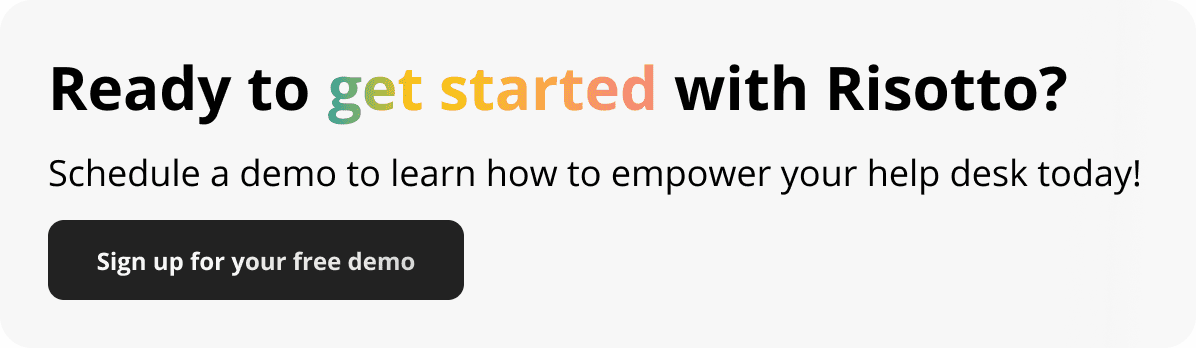
Build a more powerful help desk with Risotto
Minimize Tickets and Maximize Efficiency
Simplify IAM and Strengthen Security
Transform Slack into a help desk for every department
Schedule your free demo