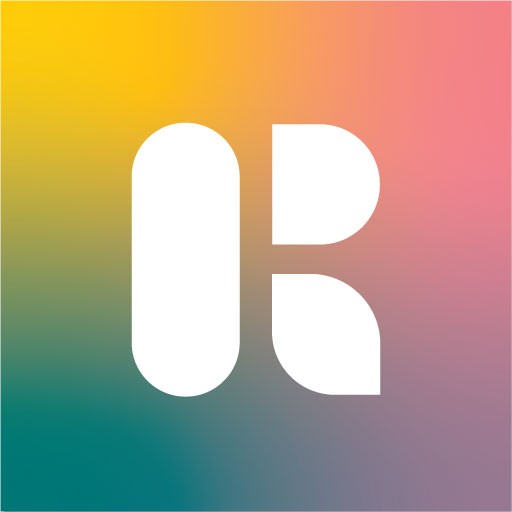
By the Risotto Team
AIOps Architecture: Streamlining IT Operations with Intelligent Automation
AIOps architecture revolutionizes IT operations by harnessing artificial intelligence and machine learning. This approach automates and enhances monitoring, incident response, and performance optimization across complex digital infrastructures. AIOps platforms integrate data from various sources, apply advanced analytics, and provide actionable insights to streamline IT management.
Organizations undergoing digital transformation can benefit significantly from AIOps architecture. It enables proactive problem detection, faster root cause analysis, and more efficient resource allocation. AIOps tools continually learn from past incidents and system behaviors, improving their accuracy and effectiveness over time.
The implementation of AIOps architecture fosters innovation in IT operations. It allows teams to shift focus from routine tasks to strategic initiatives, driving business value. By reducing manual interventions and accelerating issue resolution, AIOps contributes to improved service reliability and customer satisfaction.
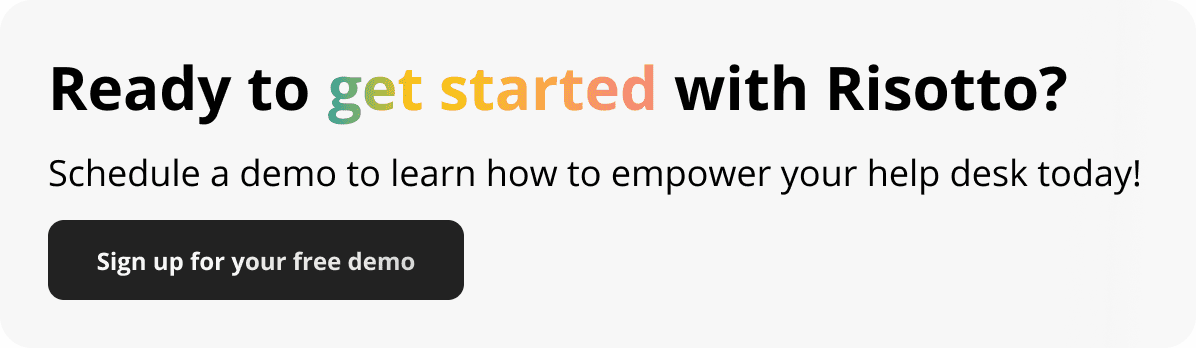
Fundamentals of AIOps Architecture
AIOps architecture integrates artificial intelligence and machine learning into IT operations. It enables organizations to automate processes, gain insights from data, and enhance overall system performance.
Architecture Principles
AIOps architecture follows key principles to ensure effective implementation. These include scalability, flexibility, and real-time processing capabilities.
Scalability allows the system to handle increasing data volumes and complexity. This is crucial as organizations grow and generate more operational data.
Flexibility enables adaptation to changing IT environments and emerging technologies. AIOps systems must integrate with various tools and platforms seamlessly.
Real-time processing is essential for rapid incident detection and response. AIOps architectures utilize stream processing and in-memory computing to achieve this.
Core Components
AIOps architectures comprise several essential components that work together to deliver intelligent IT operations.
Data ingestion and integration form the foundation. These components collect and unify data from diverse sources across the IT infrastructure.
Analytics engines process the collected data using machine learning algorithms. They identify patterns, anomalies, and potential issues.
Automation and orchestration tools execute predefined actions based on insights generated by the analytics engines. This reduces manual intervention and speeds up problem resolution.
Visualization dashboards present actionable insights to IT teams. They provide a clear view of system health, performance metrics, and potential risks.
AI-powered chatbots and virtual assistants facilitate faster communication and problem-solving. They can handle routine queries and provide guidance to IT staff.
Integrating AIOps with IT Operations
AIOps integration enhances IT operations through automated workflows and alignment with service management practices. This enables more efficient monitoring, observability, and incident response.
Operational Workflows
AIOps platforms streamline IT operations by automating routine tasks and workflows. These systems analyze data from various sources to identify patterns and anomalies. When issues arise, AIOps tools can trigger automated responses or alert relevant team members.
Integration with existing monitoring tools allows for more comprehensive observability. AIOps augments traditional monitoring by applying machine learning to detect subtle performance changes or potential problems before they escalate.
DevOps teams benefit from AIOps integration through improved collaboration and faster incident resolution. Automated root cause analysis helps pinpoint issues quickly, reducing mean time to repair (MTTR).
IT Service Management Alignment
AIOps aligns closely with IT Service Management (ITSM) practices to enhance service delivery and support. By integrating with ITSM tools, AIOps provides valuable insights for incident management, problem management, and change management processes.
Machine learning algorithms can categorize and prioritize incidents automatically, ensuring faster response times for critical issues. This integration also supports proactive problem management by identifying recurring issues and their underlying causes.
AIOps enhances change management by predicting potential impacts of planned changes. It analyzes historical data to assess risks and recommend optimal timing for implementations. This alignment between AIOps and ITSM leads to more informed decision-making and improved service quality.
Data-Driven Insights in AIOps
AIOps leverages analytics and machine learning to extract actionable insights from vast amounts of operational data. This enables more proactive and efficient IT operations management through automated anomaly detection and root cause analysis.
Data Collection and Processing
AIOps platforms ingest data from diverse sources across the IT environment. This includes performance metrics, application logs, network traffic data, and infrastructure telemetry. The data is collected in real-time and aggregated into a centralized repository.
Advanced big data technologies process and normalize the heterogeneous data streams. This creates a unified data lake for analysis. Machine learning algorithms then enrich and correlate the data to identify patterns and relationships.
Data preprocessing steps like deduplication, filtering, and feature extraction prepare the information for advanced analytics. This enables AIOps tools to handle massive volumes of operational data efficiently.
Anomaly Detection and Root Cause Analysis
AIOps uses sophisticated machine learning models to detect anomalies in IT systems. These models baseline normal behavior and flag deviations automatically. This helps identify potential issues before they impact users or services.
Anomaly detection algorithms analyze metrics, logs, and traces to spot outliers and unusual patterns. They can identify subtle changes that may indicate emerging problems.
When issues are detected, root cause analysis (RCA) techniques pinpoint the underlying sources. AIOps platforms use graph-based models and causal inference to trace dependencies and correlations across the IT stack.
RCA helps prioritize incidents and guide remediation efforts. It enables faster, more targeted responses to complex problems in dynamic environments.
AIOps Automation and Decision-Making
AIOps automation and decision-making leverage advanced analytics and machine learning to streamline IT operations. These capabilities enable rapid incident response, predictive maintenance, and proactive problem-solving.
Event Correlation and Alerting
Event correlation in AIOps identifies relationships between seemingly unrelated incidents across complex IT environments. Machine learning algorithms analyze vast amounts of data to detect patterns and anomalies.
This process reduces alert fatigue by grouping related events and suppressing redundant notifications. IT teams receive contextualized alerts with relevant information for faster troubleshooting.
Automated incident classification and prioritization ensure critical issues receive immediate attention. AIOps platforms can trigger automated remediation workflows for known issues, minimizing downtime and human intervention.
Predictive Analytics and Proactive Actions
Predictive analytics in AIOps use historical and real-time data to forecast potential issues before they impact services. Machine learning models analyze trends, seasonality, and system behaviors to identify emerging problems.
AIOps platforms can automatically initiate preventive measures based on these predictions. Examples include:
Scaling resources to handle anticipated traffic spikes
Scheduling maintenance during low-impact periods
Adjusting configurations to optimize performance
Proactive actions driven by AIOps reduce unplanned outages and improve overall system reliability. This approach shifts IT operations from reactive firefighting to strategic management of infrastructure and services.
Continuous learning and refinement of predictive models enhance accuracy over time. AIOps platforms adapt to changing environments and evolving IT landscapes, providing increasingly valuable insights and automated decision-making capabilities.
Implementing AIOps in Organizations
Successful AIOps implementation requires a strategic approach and careful consideration of organizational needs. Organizations must navigate challenges while following best practices to maximize the benefits of AIOps.
Implementation Methodology
The AIOps implementation process typically follows a phased approach. Organizations start by assessing their current IT infrastructure and identifying key areas for improvement. This assessment helps determine specific AIOps use cases and goals.
Next, organizations select appropriate AIOps tools and platforms that align with their objectives. Integration of these tools with existing systems is crucial for seamless data collection and analysis.
A pilot project often precedes full-scale implementation. This allows organizations to test AIOps capabilities, refine processes, and demonstrate value to stakeholders.
Gradual expansion of AIOps across the IT environment follows successful pilot completion. Regular evaluation and optimization ensure continued alignment with organizational goals.
Challenges and Best Practices
AIOps implementation faces several challenges. Data quality and integration issues can hinder effective analysis. Legacy systems may struggle to provide necessary data inputs. Resistance to change among IT staff can slow adoption.
To overcome these challenges, organizations should focus on data cleansing and standardization. Investing in data integration tools helps create a unified data ecosystem for AIOps.
Change management and staff training are critical. Clear communication of AIOps benefits and hands-on training sessions can boost employee engagement and adoption rates.
Establishing robust governance structures ensures proper oversight and alignment with organizational objectives. This includes defining roles, responsibilities, and decision-making processes for AIOps initiatives.
Regular performance monitoring and continuous improvement are essential. Organizations should track key metrics to measure AIOps impact on efficiency and reliability.
Frequently Asked Questions
AIOps platforms integrate advanced technologies to enhance IT operations. They leverage data analysis, machine learning, and automation to improve efficiency and service delivery.
What are the key components of an AIOps platform?
Key components of an AIOps platform include data ingestion and integration, real-time analytics, machine learning algorithms, and automation tools. These platforms also incorporate visualization capabilities and reporting features.
Event correlation engines and pattern recognition systems are essential for identifying issues across complex IT environments. Predictive analytics modules help forecast potential problems before they impact operations.
How do AIOps platforms utilize machine learning and artificial intelligence?
AIOps platforms use machine learning to analyze vast amounts of data from various IT systems. This analysis helps identify patterns, anomalies, and potential issues.
AI algorithms enable these platforms to learn from historical data and improve their accuracy over time. They can automatically categorize incidents, suggest remediation actions, and even implement solutions without human intervention.
What are the benefits of integrating AIOps into an IT environment?
Integrating AIOps into an IT environment leads to improved operational efficiency and reduced downtime. It enables faster incident resolution and proactive problem prevention.
AIOps platforms provide better visibility into complex IT infrastructures. They help organizations make data-driven decisions and optimize resource allocation.
How does AIOps contribute to IT operations management?
AIOps enhances IT operations management by automating routine tasks and providing actionable insights. It helps teams prioritize issues based on their potential impact on business operations.
These platforms enable continuous monitoring and analysis of IT systems. They facilitate faster root cause analysis and more effective capacity planning.
What factors should be considered when designing an AIOps solution?
When designing an AIOps solution, organizations should consider their existing IT infrastructure and tools. The scalability and flexibility of the platform are crucial factors.
Data quality and integration capabilities are essential for effective AIOps implementation. The solution should align with the organization's specific needs and long-term IT strategy.
How does AIOps enhance incident management and response?
AIOps enhances incident management by automating the detection and categorization of issues. It provides real-time alerts and contextual information to support teams.
These platforms can suggest or implement remediation actions based on historical data and best practices. They help reduce mean time to resolution (MTTR) and improve overall service quality.
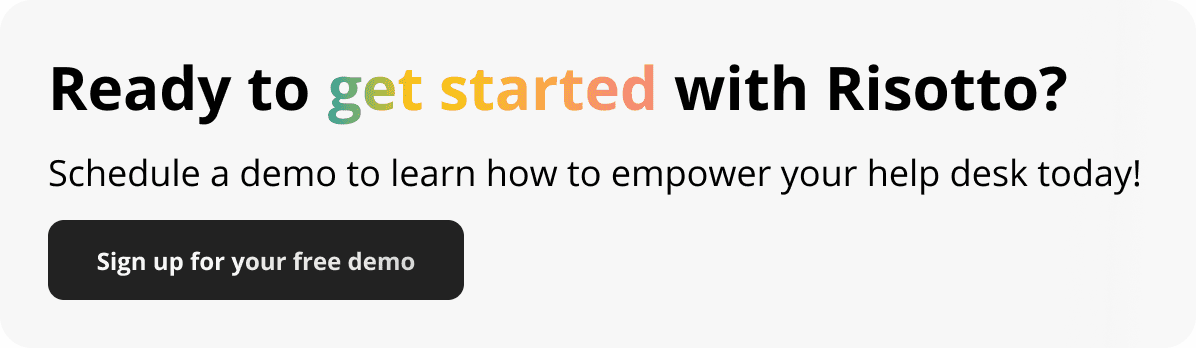
Build a more powerful help desk with Risotto
Minimize Tickets and Maximize Efficiency
Simplify IAM and Strengthen Security
Transform Slack into a help desk for every department
Schedule your free demo