IT AI: Transforming Business Operations in 2024
Artificial intelligence is transforming industries and reshaping our world. AI systems now perform complex tasks, from analyzing medical images to composing music. These innovations stem from advances in machine learning, neural networks, and data processing.
AI's capabilities are expanding rapidly, with generative AI creating original content and neural networks mimicking human cognition. This technology synthesizes vast amounts of information to produce new insights and solutions. As AI becomes more sophisticated, it promises to drive innovation across sectors.
The rise of AI raises important questions about its societal impact. While AI offers immense potential to solve problems and boost productivity, its development also requires careful consideration of ethics, privacy, and workforce changes. Ongoing research and dialogue will be crucial as AI continues to evolve.
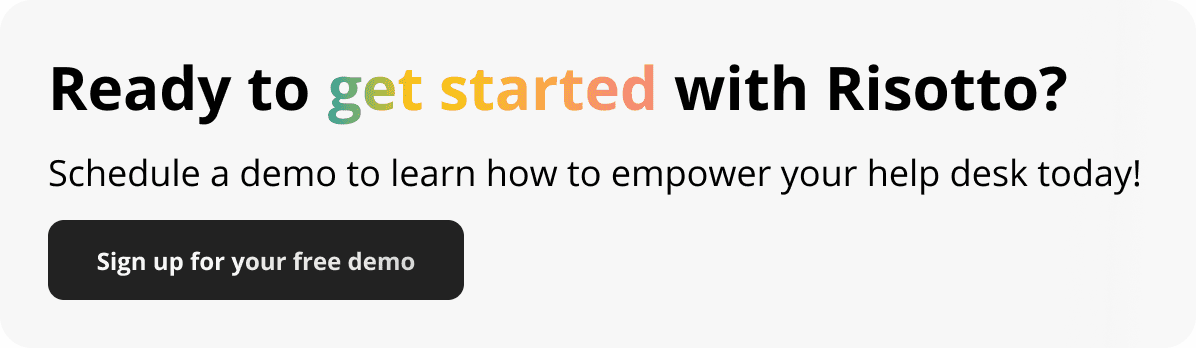
Fundamentals of Artificial Intelligence
Artificial Intelligence (AI) encompasses key concepts, evolving technologies, and complex systems like neural networks. These elements form the foundation of modern AI capabilities and applications.
Key Concepts and Terminology
AI refers to systems that mimic human intelligence. Machine Learning enables computers to improve through experience. Deep Learning, a subset of Machine Learning, uses layered neural networks to process data.
Generative AI creates new content, like images or text. Natural Language Processing allows machines to understand and generate human language.
Knowledge representation structures information for AI systems. Reasoning algorithms enable machines to draw conclusions from data.
Evolution of AI Technologies
AI has progressed from rule-based systems to more advanced approaches. Early AI relied on predefined rules and decision trees.
Machine Learning introduced algorithms that learn from data. This shift allowed AI to handle more complex tasks and adapt to new information.
Deep Learning emerged as a breakthrough, enabling AI to process vast amounts of unstructured data. It powers image recognition, speech synthesis, and language translation.
Understanding Neural Networks
Neural networks are inspired by the human brain's structure. They consist of interconnected nodes organized in layers.
Input layers receive data, hidden layers process information, and output layers produce results. Each connection has a weight that adjusts during training.
Backpropagation algorithms optimize these weights, improving network performance. Convolutional Neural Networks excel at image processing, while Recurrent Neural Networks handle sequential data like text.
AI in Everyday Life
Artificial intelligence has become deeply integrated into our daily routines, transforming how we communicate, manage tasks, and interact with technology.
From Email to Smart Devices
AI enhances email experiences by filtering spam, categorizing messages, and suggesting quick replies. Gmail's Smart Compose feature uses AI to predict and complete sentences as users type. Smart home devices leverage AI to learn user preferences and adjust settings automatically.
AI-powered cameras in smartphones recognize faces, scenes, and objects to optimize photo quality. Google Photos uses AI to organize images, making them searchable by content. Voice assistants in smart speakers use natural language processing to understand and respond to user commands.
AI-Powered Personal Assistants
Virtual assistants like Siri, Alexa, and Google Assistant use AI to perform tasks, answer questions, and control smart home devices. These assistants learn from user interactions to provide personalized recommendations and reminders.
AI-driven chatbots handle customer service inquiries on websites and messaging platforms. They analyze user input to provide relevant information and solutions. Personal finance apps use AI to track spending habits, suggest budgets, and offer financial advice.
Enhancing Productivity in Organizations
AI tools streamline workflows by automating repetitive tasks and data entry. Machine learning algorithms analyze large datasets to identify patterns and insights, supporting data-driven decision-making.
AI-powered scheduling assistants coordinate meetings by analyzing participants' calendars and preferences. Chatbots handle internal IT support requests, freeing up human staff for complex issues. AI writing assistants help employees draft emails, reports, and presentations more efficiently.
Predictive analytics tools forecast sales trends and inventory needs, optimizing supply chain management. AI-driven recruitment software screens resumes and identifies top candidates, speeding up the hiring process.
Advancements in Machine Learning
Machine learning has made remarkable strides in recent years, transforming various fields and pushing the boundaries of artificial intelligence. These advances have led to breakthroughs in game-playing, protein folding, and creative image generation.
DeepMind and AlphaGo
AlphaGo, developed by DeepMind, revolutionized the world of competitive Go in 2016. This AI system defeated world champion Lee Sedol in a historic match, showcasing its ability to master complex strategic gameplay.
AlphaGo's success stemmed from its combination of deep neural networks and reinforcement learning. It analyzed millions of expert moves and played countless games against itself to refine its strategies.
The impact of AlphaGo extended beyond Go, demonstrating the potential of AI to excel in domains requiring intuition and long-term planning. This breakthrough paved the way for further advancements in game-playing AI and strategic decision-making systems.
Alphafold and the Future of Predictive Models
DeepMind's AlphaFold represents a significant leap in protein structure prediction. This AI system can accurately determine a protein's 3D shape from its amino acid sequence, a task that previously took years of laboratory work.
AlphaFold's predictions have reached an accuracy comparable to experimental methods. Its success relies on deep learning techniques and vast databases of known protein structures.
The implications of AlphaFold are far-reaching, potentially accelerating drug discovery and enhancing our understanding of diseases. This breakthrough exemplifies how AI can tackle complex scientific challenges and open new avenues for research.
Generative AI: PARTI and Creativity
Generative AI has made significant strides in creative tasks, with systems like Google's Pathways Autoregressive Text-to-Image (PARTI) model pushing the boundaries of image generation.
PARTI can create highly detailed and coherent images from text descriptions. It employs a novel approach, breaking down the image generation process into smaller, more manageable steps.
This technology opens up new possibilities for visual content creation, from assisting artists to generating realistic simulations for various industries. The advancements in generative AI highlight the growing capability of machines to engage in tasks previously thought to require human creativity.
The Global AI Landscape
The AI landscape is rapidly evolving, with the US, EU, and UK playing pivotal roles in shaping its development. Each region brings unique strengths and approaches to AI innovation, policy, and research.
Influence of AI in the US Market
The US leads in AI innovation, driven by tech giants and startups. Google Research and OpenAI spearhead cutting-edge developments, pushing boundaries in natural language processing and machine learning. The Gemini Ecosystem exemplifies the collaborative nature of US AI research.
US companies invest heavily in AI, with applications spanning healthcare, finance, and autonomous vehicles. Silicon Valley remains a hotbed for AI talent and venture capital.
The US government has increased focus on AI policy, emphasizing national security and economic competitiveness. Debates around responsible AI use and potential regulations are ongoing.
AI Innovation and Policy in the EU
The EU takes a balanced approach to AI, emphasizing ethical considerations and data protection. The bloc's AI Act aims to regulate high-risk AI applications while fostering innovation.
European countries invest in AI research clusters and public-private partnerships. Areas of focus include industrial AI, robotics, and sustainable AI solutions.
The EU prioritizes responsible AI development, with strict data privacy laws shaping the AI landscape. This approach sometimes creates tension with rapid innovation goals.
UK's Position in AI Research
The UK maintains a strong position in AI research, leveraging its world-class universities and tech hubs. It excels in areas like deep learning and natural language processing.
Post-Brexit, the UK aims to establish itself as a global AI leader. The government has increased funding for AI research and development, focusing on areas like healthcare and climate change.
UK policymakers work to balance innovation with ethical considerations. The country's AI strategy emphasizes safety, security, and public trust in AI systems.
Ethics and Challenges in AI
AI development raises important ethical considerations and practical challenges. Addressing these issues is crucial for responsible AI implementation and societal trust.
Combating AI Biases
AI systems can perpetuate and amplify existing biases present in training data. This leads to unfair or discriminatory outcomes across various applications. Researchers and developers are working to identify and mitigate these biases through improved data collection and algorithm design.
Bias detection tools help uncover hidden prejudices in AI models. Diverse training datasets and regular audits can reduce biased outputs. Some companies implement "fairness constraints" in their AI systems to ensure equitable treatment across different demographic groups.
Ongoing challenges include balancing accuracy with fairness and addressing intersectional biases. Transparency in AI decision-making processes is essential for public trust and accountability.
AI, Privacy, and Data Security
AI systems often require vast amounts of data, raising concerns about individual privacy and data protection. Robust security measures are crucial to prevent unauthorized access or misuse of sensitive information.
Techniques like federated learning allow AI models to be trained on decentralized data, reducing privacy risks. Differential privacy adds noise to datasets, protecting individual records while maintaining overall utility.
Data governance frameworks and strict access controls help safeguard personal information used in AI systems. Encryption and secure multi-party computation enable AI analysis on sensitive data without exposing raw information.
Balancing data utility with privacy protection remains an ongoing challenge. Regulations like GDPR and CCPA set standards for data handling and user rights in AI applications.
AI in Decision Making and Governance
AI's role in high-stakes decision-making processes raises questions about accountability and transparency. In areas like criminal justice, healthcare, and finance, AI-driven decisions can significantly impact individuals' lives.
Explainable AI (XAI) techniques aim to make AI decision-making processes more interpretable. This helps identify errors, biases, and potential improvements in AI systems.
Some jurisdictions are implementing AI governance frameworks to ensure responsible deployment. These may include impact assessments, algorithmic audits, and human oversight mechanisms.
Challenges include balancing automation with human judgment and addressing potential job displacement. Ethical guidelines and industry standards are evolving to guide responsible AI use in critical sectors.
Frequently Asked Questions
AI integration in IT systems has wide-ranging implications for the industry. It impacts employment, involves various technology categories, and is driving significant transformations across many applications.
How is AI integrated into information technology systems?
AI can be integrated into IT systems through machine learning algorithms, natural language processing, and computer vision. These technologies enable automation of tasks, data analysis, and predictive capabilities. AI integration often occurs via APIs, cloud services, or custom-built solutions tailored to specific IT needs.
What are the implications of artificial intelligence for IT employment?
AI is changing the IT job landscape. Some roles may become automated, while new positions focused on AI development and maintenance are emerging. IT professionals may need to upskill to work alongside AI systems effectively.
Demand is growing for specialists in machine learning, data science, and AI engineering. Adaptability and continuous learning are becoming crucial for IT career longevity.
What are the different categories of artificial intelligence technology?
AI technologies can be categorized into several types. Machine learning uses algorithms to improve performance through experience. Deep learning employs neural networks to process complex data patterns.
Natural language processing enables computers to understand and generate human language. Computer vision allows machines to interpret and analyze visual information from the world.
How is AI expected to transform the IT industry in the coming years?
AI is set to enhance IT infrastructure management and cybersecurity. Predictive maintenance will become more prevalent, reducing system downtime. AI-powered analytics will improve decision-making processes across IT operations.
Automation of routine IT tasks will increase efficiency. Personalized user experiences and smarter help desk systems will improve customer support in IT services.
Can you list examples of AI applications in the IT sector?
AI is used in network optimization to improve performance and reduce latency. Chatbots and virtual assistants streamline IT support processes. Predictive analytics help in capacity planning and resource allocation.
AI-driven security systems detect and respond to threats more effectively. Intelligent data management systems optimize storage and retrieval processes. Code generation and debugging tools enhance software development workflows.
What are the best courses available for learning AI in the context of IT?
Top AI courses for IT professionals include "Machine Learning" by Stanford University on Coursera. "Artificial Intelligence for IT Operations" on edX provides practical insights. IBM offers "AI Engineering Professional Certificate" on Coursera.
"Deep Learning Specialization" by deeplearning.ai is highly regarded. Google's "Machine Learning Crash Course" is a solid free option. Many universities also offer specialized AI in IT programs.
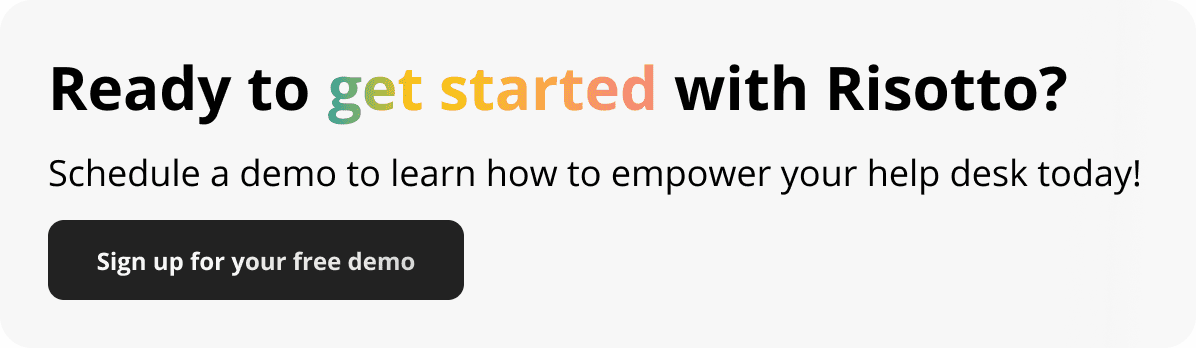
Build a more powerful help desk with Risotto
Minimize Tickets and Maximize Efficiency
Simplify IAM and Strengthen Security
Transform Slack into a help desk for every department
Schedule your free demo